- CFA Exams
- 2025 Level II
- Topic 1. Quantitative Methods
- Learning Module 5. Time-Series Analysis
Why should I choose AnalystNotes?
AnalystNotes specializes in helping candidates pass. Period.
Learning Outcome Statements PDF Download
1. Trend Models calculate and evaluate the predicted trend value for a time series, modeled as either a linear trend or a log-linear trend, given the estimated trend coefficients; describe factors that determine whether a linear or a log-linear trend should be used with a particular time series and evaluate limitations of trend models; explain the requirement for a time series to be covariance stationary and describe the significance of a series that is not stationary; | |
2. Autoregressive (AR) Time-Series Models describe the structure of an autoregressive (AR) model of order p and calculate one- and two-period-ahead forecasts given the estimated coefficients; explain how autocorrelations of the residuals can be used to test whether the autoregressive model fits the time series; explain mean reversion and calculate a mean-reverting level; contrast in-sample and out-of-sample forecasts and compare the forecasting accuracy of different time-series models based on the root mean squared error criterion; | |
3. Random Walks explain the instability of coefficients of time-series models; describe characteristics of random walk processes and contrast them to covariance stationary processes; | |
4. Unit Roots for Time-Series Analysis describe implications of unit roots for time-series analysis, explain when unit roots are likely to occur and how to test for them, and demonstrate how a time series with a unit root can be transformed so it can be analyzed with an AR model; describe the steps of the unit root test for nonstationarity and explain the relation of the test to autoregressive time-series models; | |
5. Seasonality in Time-Series Models explain how to test and correct for seasonality in a time-series model and calculate and interpret a forecasted value using an AR model with a seasonal lag; | |
6. Autoregressive Conditional Heteroskedasticity Models explain autoregressive conditional heteroskedasticity (ARCH) and describe how ARCH models can be applied to predict the variance of a time series; | |
7. Regressions with More Than One Time Series explain how time-series variables should be analyzed for nonstationarity and/or cointegration before use in a linear regression; determine an appropriate time-series model to analyze a given investment problem and justify that choice. |
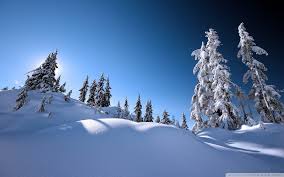
You have a wonderful website and definitely should take some credit for your members' outstanding grades.
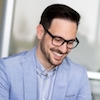
Colin Sampaleanu
My Own Flashcard
No flashcard found. Add a private flashcard for the module.
Add