- CFA Exams
- 2025 Level II
- Topic 1. Quantitative Methods
- Learning Module 4. Extensions of Multiple Regression
- Subject 1. Describe Influence Analysis and Methods of Detecting Influential Data Points
Why should I choose AnalystNotes?
Simply put: AnalystNotes offers the best value and the best product available to help you pass your exams.
Subject 1. Describe Influence Analysis and Methods of Detecting Influential Data Points PDF Download
Data observations can potentially be influential in different ways. An influential data point has a significant effect on the fitted or predicted values of the model.
If an observation has a response value that is very different from the predicted value based on a model, then that observation is called an outlier.
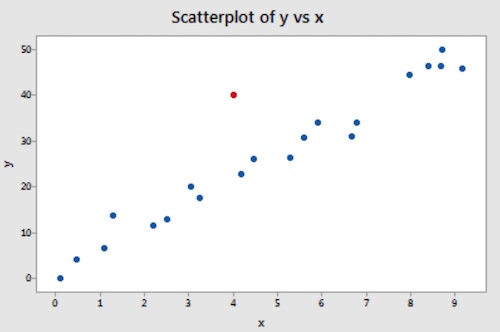
Because the red data point does not follow the general trend of the rest of the data, it would be considered an outlier.
An outlier is a point with a larger or smaller y value than the model suggests. They don't fit well with the rest of the dataset. They can either be positive or negative outliers. Positive outliers are values that are too high, while negative outliers are values that are too low.
On the other hand, if an observation has a particularly unusual combination of predictor values (e.g., one predictor has a very different value for that observation compared with all the other data observations), then that observation is said to have high leverage.
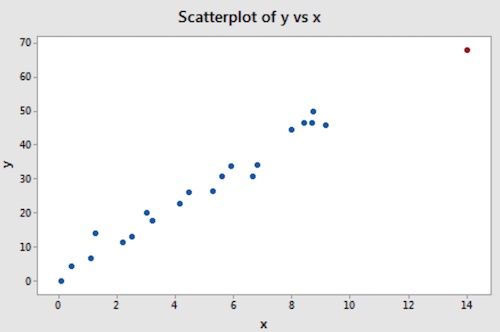
In this case, the red data point does follow the general trend of the rest of the data. Therefore, it is not deemed an outlier here. However, this point does have an extreme x value, so it does have high leverage.
High-leverage points have a relatively large influence on the fitted values of the regression line. This means that if you were to remove a high-leverage point from your dataset, the regression line would change quite a bit.
Thus, there is a distinction between outliers and high leverage observations, and each can impact our regression analyses differently. It is also possible for an observation to be both an outlier and have high leverage.
Methods of Detecting Influential Data Points
Leverage
A measure for identifying a high-leverage point is leverage. Leverage, hii, is a measure of how far away an individual data point is from the mean of the rest of the data. For a particular independent variable, leverage measures the distance between the ith observation of that variable and the mean of the variable across n observations.
Leverage lies between 0 and 1. The sum of individual leverages for all observations is k + 1, where k is the number of independent variables.
If leverage is greater than 3 ( k + 1 _ n ) , where k is the number of independent variables, then the observation is potentially influential.
Studentized Residual
A measure for identifying an outlier is studentized residuals. The basic idea is to delete the observations one at a time, each time refitting the regression model on the remaining n-1 observations. Then, we compare the observed response values to their fitted values based on the models with the ith observation deleted. This produces deleted residuals. Standardizing the deleted residuals produces studentized residuals.
When interpreting the studentized residual, it is important to remember that a zero value indicates that the data point is not an outlier. Positive values indicate that the data point is above the regression line, while negative values indicate that the data point is below the regression line. Generally speaking, a studentized residual should be considered significant if it is greater than 2 in absolute value.
A more reliable way: If the studentized residual is greater than the critical value of the t-statistic with n - k - 2 degrees of freedom, then the observation is potentially influential.
Cook's Distance
Di is used in Regression Analysis to find influential outliers in a set of predictor variables. It measures how much the estimated values of the regression change if observation i is deleted. The measurement is a combination of each observation's leverage and residual values; the higher the leverage and residuals, the higher the Cook's distance.
Data points with a Cook's distance greater than 1 or 2 (k/n)1/2 are considered to be influential. This means they can potentially skew the results of the analysis.
User Contributed Comments 0
You need to log in first to add your comment.
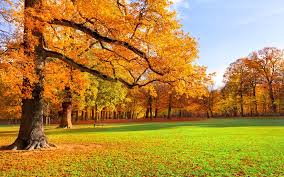
Thanks again for your wonderful site ... it definitely made the difference.
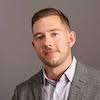
Craig Baugh
My Own Flashcard
No flashcard found. Add a private flashcard for the subject.
Add