- CFA Exams
- 2025 Level II
- Topic 1. Quantitative Methods
- Learning Module 3. Model Misspecification
- Subject 2. Heteroskedasticity
Why should I choose AnalystNotes?
AnalystNotes specializes in helping candidates pass. Period.
Subject 2. Heteroskedasticity PDF Download
Recall that the 2nd assumption of classical normal multiple linear regression model is homoscedasticity - The variance of the error term is the same for all values of the independent variables. The word homoscedastic is derived from two Greek words that mean "having the same spread." If the error terms do not have equal variances, they are said to be heteroscedastic, from two Greek words meaning "having different spreads".
For example, suppose we have data on the annual income and annual consumption expenditures of individual families, and we formulate a model in which consumption expenditure are explained as a function of income. In this case, the assumption of homoscedastic may not be plausible because we would expect less variation in consumption for low-income families than for high-income families.
- At low incomes, the typical level of consumption is low and the variation about this level is relatively small. Consumption cannot fall too far below the average level, because this would mean near starvation for the family. In addition, consumption cannot rise too far above the average, because the family's assets and credit position would not allow it.
- The above constraints are generally less binding for families with higher incomes.
The appropriate model in this case would contain heteroscedastic error terms.
If the errors do not have equal variances, the least-squares estimates b0, b1, ..., bk will still be unbiased estimates of the population parameters β0, β1, ... βk, but the estimates will not be efficient. In addition, the least-squares estimate of σe2 and the estimates of the variances of the estimated coefficient bk will be biased. If we know the nature of the heteroscedasticity, there exists an alternative linear unbiased estimator that yields estimates whose variances are smaller than the least-squares variances.
The fact that all the estimated variances are biased invalidates all the t tests and F tests used to test hypotheses about the values of the population parameters and invalidates all the confidence intervals for the population parameters.
Although heteroscedasticity can cause problems for statistical inference in the linear regression model, not all types of heteroscedasticity affect statistical inference.
- Unconditional heteroscedasticity occurs when the heteroscedasticity is NOT correlated with the independent variables in the multiple regression. Although this form of heteroscedasticity violates one assumption of the linear regression model, it creates no major problems for statistical inference.
- Conditional heteroscedasticity occurs when the heteroscedasticity is correlated with (conditional on) the values of the independent variables in the multiple regression.
A popular test for conditional heteroskedasticity is the Breusch-Pagan test, which involves regressing the squared residuals upon the independent variables within the regression. If no conditional heteroskedasticity is present, then the independent variables will not explain much of the variation of the squared residuals.
Conversely, if the regression suffers from conditional heteroskedasticity, then the independent variables will explain a significant portion of the variation of the squared residuals. The null and alternate hypotheses of the Breusch-Pagan test for conditional heteroskedasticity bias are as follows:
- H0: no conditional heteroskedasticity exists within the regression residuals.
- H1: conditional heteroskedasticity exists within the regression residuals.
Given a null hypothesis of no conditional heteroskedasticity, Breusch and Pagan showed that [n x R2] will be a Chi-squared random variable with k degrees of freedom. The test statistic for the regression is "(n x R2)," in which both values are taken from the regression of the squared residuals upon the underlying independent variables. If the test statistic is greater than the critical value provided from the Chi-squared distribution with k degrees of freedom, then the null hypothesis of no conditional heteroskedasticity is rejected.
Fortunately, correcting for conditional heteroskedasticity is relatively simplistic, and many statistical software programs automatically search out and correct for conditional heteroskedasticity. There are two methods to correct for conditional heteroskedasticity.
The first method involves the computation of robust standard errors. It corrects the standard errors of the linear regression model's estimated parameters to account for the conditional heteroskedasticity. These robust standard errors can be calculated using many statistical software programs, and quantitative analysts are strongly recommended to utilize this function if the results of a regression indicate conditional heteroskedasticity. Manual calculation of robust standard errors is functionally complex, and beyond the scope of the CFA exam.
The second method to correct for conditional heteroskedasticity, generalized least squares, modifies the original regression equation in an attempt to eliminate the conditional heteroskedasticity. Utilizing generalized least squares is simple with the aid of a statistical software package, and many programs offer this function. However, the manual use of generalized least squares is essentially a trial-and-error process. Further, the manual decomposition of generalized least squares is beyond the scope of the CFA exam. What is important is that you are able to identify heteroskedasticity bias and recognize the two methods for correcting conditional heteroskedasticity bias.
User Contributed Comments 3
User | Comment |
---|---|
danlan2 | Two methods: robust standard errors and generalized least squares. The first corrects the standard errors and the second modifies the original regression equation. |
danlan2 | Two types: unconditional and conditional (Breusch-Pagon test). |
nishikori | Robust Standard Errors AKA White-corrected standard errors |
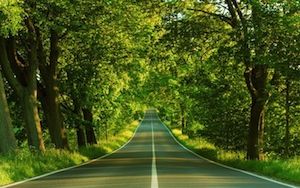
I am using your study notes and I know of at least 5 other friends of mine who used it and passed the exam last Dec. Keep up your great work!
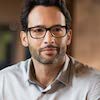
Barnes
My Own Flashcard
No flashcard found. Add a private flashcard for the subject.
Add